Status
Completed
Title
Development and evaluation of a tool for predicting risk of short-term adverse outcomes due to COVID-19 in the general UK population
What were the objectives of the study?
Introduction
The first UK cases of the novel coronavirus infection that causes COVID-19 were confirmed on 24th January 2020 and the first UK death on 28th Feb 2020. Since then the virus has spread rapidly. There are currently no vaccines or treatments which have been proven to be effective and safe for COVID-19. The government has therefore used social distancing and other methods to limit the increase in cases and protect the most vulnerable.
Early on, the government made a decision to try to protect those individuals thought to be at greatest risk of severe COVID-19 outcomes. As a result, advice was tailored to three different groups in the population:
● Clinically Extremely Vulnerable (CEV) group: who were advised to ‘shield’. This means staying at home at all times and avoiding all face-to-face contact for a period of at least 12 weeks.
● Clinically Vulnerable (CV) group: who were advised to follow stringent social distancing measures.
● The remainder of the population: who follow mandatory social distancing measures; lockdown (23rd March-11th May) followed by gradual easing of measures.
The clinically vulnerable group was identified by doctors and expert groups, largely based on groups who were eligible to receive a flu-vaccine. Within this group, certain individuals were advised to shield (the CEV group) because it was believed their medical condition may put them at even higher risk of becoming very unwell or dying with COVID-19 if they became infected.
The original shielded list was developed early in the outbreak and people on it were identified by a variety of methods. The aim was that the list would be adapted as our knowledge of the COVID-19 improved.
As we learn more about Covid-19, it is clear that some people are more likely than others to become very unwell, requiring hospitalisation, or die if they become infected. We know that factors like age, sex, ethnicity, weight, occupation, certain medical conditions and being on certain medication may have an impact both on the chance of getting the virus, and of being hospitalised and dying if infected.
England’s Chief Medical Officer has therefore asked this expert group to consider the latest evidence on risk factors for severe COVID-19 outcomes and death, and assess whether it is possible to create a calculator that takes into account these risk factors.
Risk calculators have been developed in the past, to individualise prevention or treatment strategies for many conditions. For example QRisk, which is a well-established cardiovascular disease (CVD) risk score, has been in use across the NHS since 2009. The score estimates the risk of a person developing CVD over the next 10 years. It is designed for doctors to monitor and identify people at high risk of developing CVD for so that action can be taken to reduce their risk of developing CVD.
Objective
Our objective is to develop a risk calculator that will enable patients, clinicians and the public to understand their risk of being infected by, and then dying (or requiring hospitalisation) from, COVID-19 based on their risk factors and circumstances, such as age, gender, ethnic group, longstanding illnesses, and current medications.
Example use cases
A risk calculator could be used in the following ways:
● By policymakers, in dividing the whole population into groups with similar levels of risk, and then deciding what the shielding and social distancing advice should be to these groups going forwards.
● By doctors, to help patients understand their risk and the steps they can take to modify their risk (such as changing lifestyle behaviours, or prioritising giving a vaccine if and when one is developed).
● By individuals, to understand the government advice for their level of risk, and allow them to make more informed decisions about the level of risk they are willing to tolerate in their daily lives, which is largely related to the number of contacts a person has.
How was the research done?
Methods
Our study will use electronic health records to understand the factors that are related to the risk of becoming infected with, hospitalised, and dying from COVID-19. Electronic health records are routinely collected in GP databases and hospitals whenever a person registers or uses a service. The different data sources can be linked together, along with other sources of data such as the information recorded on death certificates. Patient identifiable information (such as name, date of birth, and address) can be removed, which protects the privacy of individual patients.
We will use such a linked dataset called the QResearch database, which was established in 2002 and has been used for similar research into risk prediction calculator, for example the QRisk calculator. The database collects data from 1205 GP practices. This will be linked to other data sources such as lab reports of COVID-19 positive test results.
It is important to consider a wide range of factors to enable us to develop a calculator that is able to distinguish well between people with different levels of risk. The analysis will calculate the impact of each of these factors, when considered together, on the risk of being infected by, and then dying from COVID-19, while accounting for any data that is missing. Once the tool is developed, its performance will be tested using alternative linked datasets to see if it is accurate in predicting risk of getting COVID-19 and dying of it. In the finished calculator, users can enter values for the above risk factors to calculate a risk score.
Strengths and weaknesses
Key strengths include the large sample of patients included, which is representative of the UK population, and the wealth of data on different risk factors and outcomes achieved through data linkage. Limitations include the potential for issues around testing, diagnosis, and missing data to influence and skew the results, although where possible, steps will be taken to address these. To ensure full transparency, the equations behind our risk prediction tool will be published in full and freely available, to allow other researchers to assess it and use it.
Chief Investigator
Professor Julia Hippisley-Cox, Nuffield Department of Primary Care Health Sciences, University of Oxford
Lead Applicant Organisation Name
Sponsor
University of Oxford
Location of research
University of Oxford
Date on which research approved
08-Jun-2020
Project reference ID
OX100
Generic ethics approval reference
18/EM/0400
Are all data accessed are in anonymised form?
Yes
Brief summary of the dataset to be released (including any sensitive data)
From the dataset, we will include patients aged 0-100 years between 24th January and May 2020. Then we will look at the following factors to see if they are related to risk of being infected with and dying from COVID-19:
● Demographic factors (such as age, gender, ethnicity and level of deprivation)
● Lifestyle factors (such as smoking status)
● Conditions currently included on the shielded patient list
● Other longstanding illnesses (such as diabetes, high blood pressure, cancer)
● Current medication
What were the main findings?
We have developed and evaluated a novel clinical risk prediction model (QCOVID) to estimate risks of hospital admission and mortality due to covid-19. We have used national linked datasets from general practice and national SARS-CoV-2 testing, death registry, and hospital episode data for a sample of more than 8 million adults representative of the population of England. The risk models have excellent discrimination. Although the calibration for the hospital admission outcome was good in both time periods, some under-prediction existed for the mortality outcome in the second validation cohort, which improved after recalibration. The recalibration method could be used to transport the risk models to other settings or time periods with different absolute risks of covid-19.
QCOVID represents a new approach for risk stratification in the population. It could also be deployed in several health and care applications, either during the current phase of the pandemic or in subsequent “waves” of infection (with recalibration as needed). These could include supporting targeted recruitment for clinical trials, prioritisation for vaccination, and discussions between patients and clinicians on workplace or health risk mitigation—for example, through weight reduction as obesity may be an important modifiable risk factor for serious complications of covid-19 if a causal association is established.
Although QCOVID has been specifically designed to inform UK health policy and interventions to manage covid-19 related risks, it also has international potential, subject to local validation. One of the variables in our model (the Townsend measure of deprivation) may need to be replaced with locally available equivalent measures, or some recalibration may be needed. Previous risk prediction models based on QResearch have been validated internationally and found to have good performance outside of the UK
Funding Source
NIHR Program Grant for Applied Research
Public Benefit Statement
Research Team
- Dr Ash K. Clift, Nuffield Department of Primary Care Health Sciences, University of Oxford
- Professor Carol Coupland, Division of Primary Care, University of Nottingham
- Professor Ruth Keogh, Faculty of Epidemiology and Population Health, London School of Hygiene & Tropical Medicine
- Dr Karla Diaz-Ordaz, Faculty of Epidemiology and Population Health, London School of Hygiene & Tropical Medicine
- Dr Elizabeth Williamson, Faculty of Epidemiology and Population Health, London School of Hygiene & Tropical Medicine
- Professor Ewen M. Harrison, Division of Health Sciences, University of Edinburgh
- Professor Andrew Hayward, Institute ofEpidemiology and Health Care, University College London
- Professor Harry Hemingway, The UCL Institute for Health Informatics, University College London.
- Professor Peter Horby, Nuffield Department of Medicine, University of Oxford
- Dr Nisha Mehta, Office of the Chief Medical Officer, Department of Health & Social Care
- Professor Jonathan Benger, NHS Digital, 1 Trevelyan Square, Leeds
- Professor Kamlesh Khunti, Leicester Diabetes Centre, University of Leicester
- Professor Aziz Sheikh, Usher Institute, University of Edinburgh
- Professor Jonathan Valabhji, National Clinical Director for Diabetes & Obesity, NHS England
- Professor Ronan A. Lyons, Health Data Research UK, Swansea University
- Dr John Robson, Queen Mary’s University, London
- Professor Calum Semple, Institute of Translational Medicine, University of Liverpool
- Professor Frank Kee, Centre for Public Health, Queen’s University Belfast
- Professor Peter Johnson, National Clinical Director for Cancer, NHS England & NHS Improvement
- Professor Susan Jebb, Nuffield Department of Primary Care Health Sciences
- Dr Tony Williams, Working Fit Ltd
Plans for use and dissemination
We plan to publish our research protocol, and the resulting risk prediction model, in peer-reviewed journals. The analysis will be presented to Government scientific advisors to inform the next stage of the UK COVID-19 response. While our aim is primarily to produce a tool to inform the COVID-19 response in the UK, our methods, analysis and findings may be informative to other countries and their responses to the pandemic
More information is available on the University of Oxford, Nuffield Department of Primary Care Health Sciences website.
Please see here for how NHS DIgitial is using the QCOVID tool’
https://digital.nhs.uk/coronavirus/risk-assessment#how-we-re-using-qcovid
Approval Letter
Publications
-
Development and evaluation of a tool for predicting risk of short-term adverse outcomes due to COVID-19 in the general UK population; Research Protocol
Authors: Julia Hippisley-Cox, Ash K. Clift, Carol Coupland, Ruth Keogh, Karla Diaz-Ordaz, Elizabeth Williamson, Ewen M. Harrison, Andrew Hayward, Harry Hemingway, Peter Horby, Nisha Mehta, Jonathan Benger, Kamlesh Khunti, David Spiegelhalter, Aziz Sheikh, Jonathan Valabhji, Ronan A. Lyons, John Robson, Calum Semple, Frank Kee, Peter Johnson, Susan Jebb, Tony Williams, David Coggon
Ref:
https://www.qresearch.org/media/1202/ox100-covid-19-risk-prediction-tool-112.pdf -
Living risk prediction algorithm (QCOVID) for risk of hospital admission and mortality from coronavirus 19 in adults: national derivation and validation cohort study
Authors: Clift AK, Coupland CAC, Keogh RH, Karla Diaz-Ordaz K, Williamson E, Harrison EM Hayward A, Harry H, Horby P, Mehta N, Benger J, Khunti K, Spiegelhalter D, Sheikh A, Valabhji J, Lyons RA, Robson J, Semple MG, Kee F, Johnson P, Jebb S, Williams T, Hippisley-Cox J
Ref: 2020;371:m3731
https://doi.org/10.1136/bmj.m3731 -
Protocol for the development and evaluation of a tool for predicting risk of short-term adverse outcomes due to COVID-19 in the general UK population
Authors: Hippisley-Cox J, Clift AC, Coupland CAC, Keogh R, Diaz-Ordaz K, Williamson E, Harrison E, Hayward A, Hemingway H, Horby P, Mehta N, Benger JK, Khunti K, Spiegelhalter D, Sheikh A, Valabhji J, Lyons RA, John Robson J, Gracie Semple M, Kee F, Johnson P, Jebb S, Tony Williams T, Coggon D
Ref:
https://www.medrxiv.org/content/10.1101/2020.06.28.20141986v1 -
Living risk prediction algorithm (QCOVID) for risk of hospital admission and mortality from coronavirus 19 in adults: national derivation and validation cohort study. BMJ 2020 (letter)
Authors: Clift A, Semple MG, Khunti K, Coupland C, Sheikh A, Valabhji J & Hippisley-Cox J.
Ref: Ref1
https://www.bmj.com/content/371/bmj.m3731/rr-4 -
An external validation of the QCovid risk prediction algorithm for risk of mortality from COVID-19 in adults: national validation cohort study in England (pre-print)
Authors: Vahe Nafilyan, Ben Humberstone, Nisha Metha, Ian Diamond, Luke Lorenzi, Piotr Pawelek, Ryan Schofield, Jasper Morgan, Paul Brown, Ronan Lyons, Aziz Sheikh, Julia Hippisley-Cox
Ref:
https://www.medrxiv.org/content/10.1101/2021.01.22.21249968v1 -
An external validation of the QCovid risk prediction algorithm for risk of mortality from COVID-19 in adults: a national validation cohort study in England
Authors: Vahé Nafilyan, BenHumberstone, Nisha Mehta, Prof Ian Diamond, Prof Carol Coupland, Luke Lorenzi Piotr Pawelek, Ryan Schofield, Jasper Morgan, Paul Brown Prof Ronan Lyons, Prof Aziz Sheikh, Prof Julia Hippisley-Cox
Ref:
https://www.sciencedirect.com/science/article/pii/S2589750021000807 -
Sickle-cell disorders and severe COVID-19 outcomes: a cohort study
Authors: Clift AK, Saatci D, Coupland CA, Dambha-Miller H, Hippisley-Cox J
Ref:
https://www.acpjournals.org/doi/10.7326/M21-1375 -
Risk prediction of covid-19 related death and hospital admission in adults after covid-19 vaccination: national prospective cohort study
Authors: Julia Hippisley-Cox 1, Carol Ac Coupland 2 3, Nisha Mehta 4, Ruth H Keogh 5, Karla Diaz-Ordaz 5, Kamlesh Khunti 6, Ronan A Lyons 7, Frank Kee 8, Aziz Sheikh 9, Shamim Rahman 10, Jonathan Valabhji 11, Ewen M Harrison 9, Peter Sellen 10, Nazmus Haq 10, Malcolm G Semple 11, Peter W M Johnson 12, Andrew Hayward 13, Jonathan S Nguyen-Van-Tam 3 10
Ref:
https://pubmed.ncbi.nlm.nih.gov/34535466/ -
External validation of the QCovid risk prediction algorithm for risk of COVID-19 hospitalisation and mortality in adults: national validation cohort study in Scotland
Authors: Colin R Simpson, Chris Robertson, Steven Kerr, Ting Shi, Eleftheria Vasileiou, Emily Moore, Colin McCowan, Utkarsh Agrawal, Annemarie Docherty, Rachel Mulholland, Josie Murray, Lewis Duthie Ritchie, Jim McMenamin, Julia Hippisley-Cox, Aziz Sheikh
Ref:
https://thorax.bmj.com/content/early/2021/11/14/thoraxjnl-2021-217580.abstract?ct= -
QCOVID in Scotland: time to recalibrate our expectations?
Authors: Rishi K Gupta, Maarten van Smeden
Ref:
https://thorax.bmj.com/content/early/2021/11/14/thoraxjnl-2021-218169.full?ct= -
Validating the QCOVID risk prediction algorithm for risk of mortality from COVID-19 in the adult population in Wales, UK
Authors: Jane Lyons, Vahé Nafilyan, Ashley Akbari, Gareth Davies, Rowena Griffiths, Ewen Harrison, Julia Hippisley-Cox, Joe Hollinghurst, Kamlesh Khunti, Laura North, Aziz Sheikh, Fatemeh Torabi, Ronan Lyons
Ref:
https://ijpds.org/article/view/1697/ -
Common protocol for validation of the QCOVID algorithm across the four UK nations
Authors: Steven Kerr, Chris Robertson, Vahe Nafilyan, Ronan A Lyons, Frank Kee, Christopher R Cardwell, Carol Coupland, Jane Lyons, Ben Humberstone, Julia Hippisley-Cox, Aziz Sheikh
Ref:
https://bmjopen.bmj.com/content/12/6/e050994.abstract?ct= -
An external validation of the QCOVID3 risk prediction algorithm for risk of hospitalisation and death from COVID-19: An observational, prospective cohort study of 1.66m vaccinated adults in Wales, UK
Authors: Lyons J, Nafilyan V, Akbari A, Bedston S, Harrison E, Hayward A, Hippisley-Cox J, Kee F, Khunti K, Rahman S, Sheikh A, Torabi F, Lyons RA
Ref:
https://journals.plos.org/plosone/article?id=10.1371/journal.pone.0285979 -
Risk prediction of covid-19 related death or hospital admission in adults testing positive for SARS-CoV-2 infection during the omicron wave in England (QCOVID4): cohort study
Authors: Hippisley-Cox J, Khunti K, Sheikh A, Nguyen-Van-Tam JS, Coupland CAC
Ref:
https://www.bmj.com/content/381/bmj-2022-072976 -
Dynamic updating of clinical survival prediction models in a changing environment
Authors: Tanner KT, Keogh RH, Coupland CAC, Hippisley-Cox J & Diaz-Ordaz K
Ref:
https://diagnprognres.biomedcentral.com/articles/10.1186/s41512-023-00163-z -
QCOVID Risk Prediction Tool Final Report to NIHR Policy Research Programme
Authors: Julia Hippisley-Cox, Carol AC Coupland, Nisha Mehta, Ruth H Keogh, Karla Diaz-Ordaz, Kamlesh Khunti, Ronan A Lyons, Frank Kee, Aziz Sheikh, Sharon Dixon, Shamim Rahman, Jonathan Valabhji, Ewen M Harrison, Peter Sellen, Nazmus Haq, Malcolm G Semple, Peter WM Johnson, Andrew Hayward, Jonathan S Nguyen-Van-Tam
Ref:
https://www.qresearch.org/media/qarlvbvv/qcovid-final-report-nihr-may-2024.pdf -
Uptake and safety of Sotrovimab for prevention of severe COVID-19 in a cohort and self-controlled case series study
Authors: Patone M, Snelling AJHL, Tibble H, Coupland C, Sheikh A, Hippisley-Cox J
Ref:
https://www.nature.com/articles/s43856-024-00720-7?utm_source=rct_congratemailt&utm_medium=email&utm_campaign=oa_20250116&utm_content=10.1038/s43856-024-00720-7
Press Releases
- Development and evaluation of a tool for predicting risk of short-term adverse outcomes due to COVID-19 in the general UK population; Press Release
- COVID-19 is 10 times deadlier for people with Down syndrome, raising calls for early vaccination
- Oxford-led technology to help those at high risk from Covid-19
- Model that predicts patient risk from COVID-19 wins Florence Nightingale Award for Excellence in Healthcare Data Analytics
- COVID-19 risk prediction tool wins national award
- QCovid highly commended for ‘best use of technology in Patient Safety’ at the 2021 HSJ Patient Safety Awards
- COVID-19: QCovid tool's new algorithm identifies those most at-risk from coronavirus after vaccination
- New tool identifies groups most at-risk from Covid after vaccination
- Covid travel news: Government to introduce charges for tests, as bookings surge after rules eased
- People with chronic conditions among most at risk from Covid even after jabs
- Researchers identify vaccinated patient groups most at risk of serious Covid
- Older people and men 'more likely to suffer' with Covid even after two jabs
- Men 'more likely' to face severe outcomes following double-vaccination, warns new study
- Calculator reveal which vaccinated Brits are most at risk of dying from Covid
- Researchers unveil algorithm to identify COVID-19 patients at high risk despite vaccination
- People with Down's syndrome and severe kidney disease are most at risk of being admitted to hospital or death after having two Covid vaccines, study finds
- Age, illness and vaccine status still biggest factors as new Covid calculator shows who’s most at risk
- Vaccinated groups at highest risk of Covid-19 hospitalisation and death identified using new QCovid tool
- Hippisley-Cox wins John Perry Prize for pseudonymisation tools made available by QResearch (29 October 2013)
- Covid research partnership wins national computing innovation award
- Our Open Access Publication of the Month - January 2022
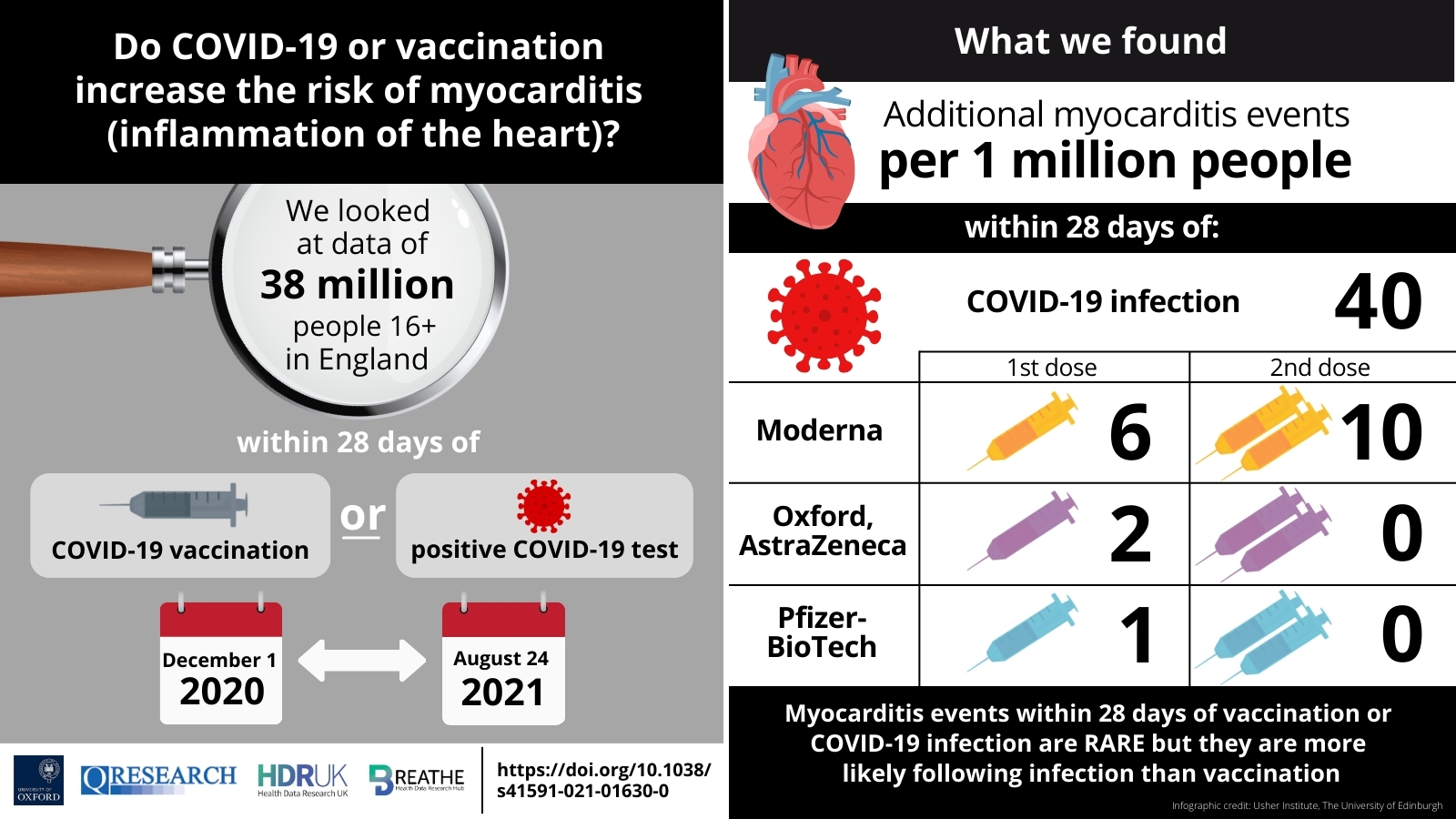
Access Type
Trusted Research Environment (TRE)